In Industry 4.0, there is a need for automated diagnosis of process monitoring alarms along with root cause determination and process adjustment. Computationally intensive and adaptable approaches such as those incorporated into ProcessMiner software are required for these reasons.
Our readers are likely familiar with the industrial practice being broken down into the following four roughly defined eras: Industry 1.0 (use of water and steam power and mechanization starting about 1800), Industry 2.0 (use of electricity, mass production, and assembly lines starting about 1900), Industry 3.0 (use of automation and information technology starting about 1970) and now Industry 4.0 (use of cyber-physical control and big data starting about 2011). In addition to advances in technology, these eras of industrial practice could also be characterized in terms of increases in the availability and use of data.
Industry 2.0 and Statistical Process Monitoring
Statistical process monitoring (SPM) originated with Walter Shewhart’s work at Western Electric during the Industry 2.0 era. Shewhart was the first person to recognize that the time order of industrial data could be very informative. Data collection was slow, manual, and expensive in his day. Sampling frequencies were typically low. The first Shewhart chart in 1924, for example, involved a plot of proportions of non-conforming items with the data aggregated over months. Any calculations had to be simplified in order to be done by hand. The Shewhart control limits were set at plus and minus three standard deviations of the control chart statistic from the centerline. The Western Electric Handbook, which operationalized Shewhart’s ideas, was first published in 1956.
There were some major advances in SPM methods during Industry 2.0. Harold Hotelling introduced the first chart for monitoring multivariate data in 1947. S. W. Roberts introduced the exponentially weighted moving average (EWMA) chart in 1959 and E. S. Page introduced the cumulative sum (CUSUM) chart in 1961. These methods made better use of information in recent data. It does not seem, however, that the EWMA and CUSUM methods had much of an influence on industrial practice in this era.
Richard Freund of Kodak introduced the acceptance control chart in 1957. The purpose of his chart was to widen the Shewhart chart control limits to avoid the detection of process shifts that were considered to be too small to be of practical importance.
As another notable contribution, in 1959 J. E. Jackson proposed a multivariate monitoring method based on the use of principal components. It was much later, however, before the principal component methods could be used to their full potential.
Industry 3.0 and Statistical Process Monitoring
The quality revolution in the U.S. started around 1980, well into the Industry 3.0 era. The primary driving force behind the quality revolution was quality and price competition from Japanese companies. Statistical monitoring methods became widely used and in some cases oversold. W. E. Deming was the de facto spiritual leader of the quality revolution because much of the Japanese quality success was attributed to his teaching in Japan in 1950 and later. Deming was a strong proponent of process improvement by continual variation reduction. He also advocated the use of the Shewhart control chart, writing that no method of monitoring was better. In particular, he was opposed to the widening of the Shewhart three-sigma control limits.
As the amount of data increased during Industry 3.0, more and more SPM methods were developed. Computation became much less of an issue with software, such as Minitab® and JMP®, becoming available. There were additional multivariate methods introduced such as the multivariate EWMA chart and multivariate CUSUM charts. As sampling frequencies increased, it became important to account for autocorrelation in the data. Manufacturing processes are almost invariably multi-stage, so methods were developed to account for incoming quality, not just outgoing process quality, at each stage.
Methods were developed in Industry 3.0 for monitoring processes where there was more than just the one variance component assumed with Shewhart control charts. For example, with batch data, there could be variation between batches as well as variation within batches. There were extensions required for the monitoring of functional data (called profile monitoring), where quality is best characterized by a relationship between a response variable and one or more explanatory variables. One of the first applications of profile monitoring was developed at the U.S. National Bureau of Standards for monitoring calibration curves. Some methods were also developed for monitoring image and video data.
Industry 4.0 and Statistical Process Monitoring
Although standard Industry 2.0 and 3.0 statistical process monitoring methods can remain useful, they fail to work well, if at all, in many Industry 4.0 applications. Industry 4.0 data are often high-dimensional with high sampling frequencies. Control charts have been developed to signal for data that indicated a statistically significant process change. With massive amounts of data, any change, however small, becomes statistically significant. In an increasing number of cases, the focus needs to be on detecting, to the extent possible, only process changes of practical importance. In effect, control charts and warning systems, in general, need to be tuned to detect shifts of practical interest, which will vary by application.
Having a smoke alarm that is activated by the striking of a match would be very undesirable in a kitchen, for example, but such sensitivity might be desirable in an airplane lavatory. Thus, there needs to be a return to some of the basic ideas of Richard Freund and the acceptance control chart. The focus needs to be on the practical importance, not just the statistical significance, of process changes.
There are other complicating issues that can arise with Industry 4.0 data. The use of sensor technology leads to high dimensional data, so dimension reduction methods can be helpful to take advantage of the resulting duplication of information. The high sampling frequencies require decisions about the appropriate level of data aggregation. Sampling frequencies will rarely be synchronized for the measured variables, as assumed in Industry 3.0 multivariate methods. In particular, quality variables are often measured at much lower frequencies than process variables and are often characterized by delays such as those due to lab work.
The types of data in Industry 4.0 can vary as well with attribute data, variables data, profiles and image data all required for decision making. In addition, there can be complicated relationships between variables involving correlation and causation.
The data involved in Industry 4.0 applications can be far too complicated to be handled by traditional statistical process monitoring approaches. Modeling and using the process data effectively, even by process experts, is often impossible. In Industry 4.0 there is a need for automated diagnosis of process monitoring alarms along with root cause determination and process adjustment. Computationally intensive and adaptable approaches such as those incorporated into ProcessMiner software are required for these reasons.
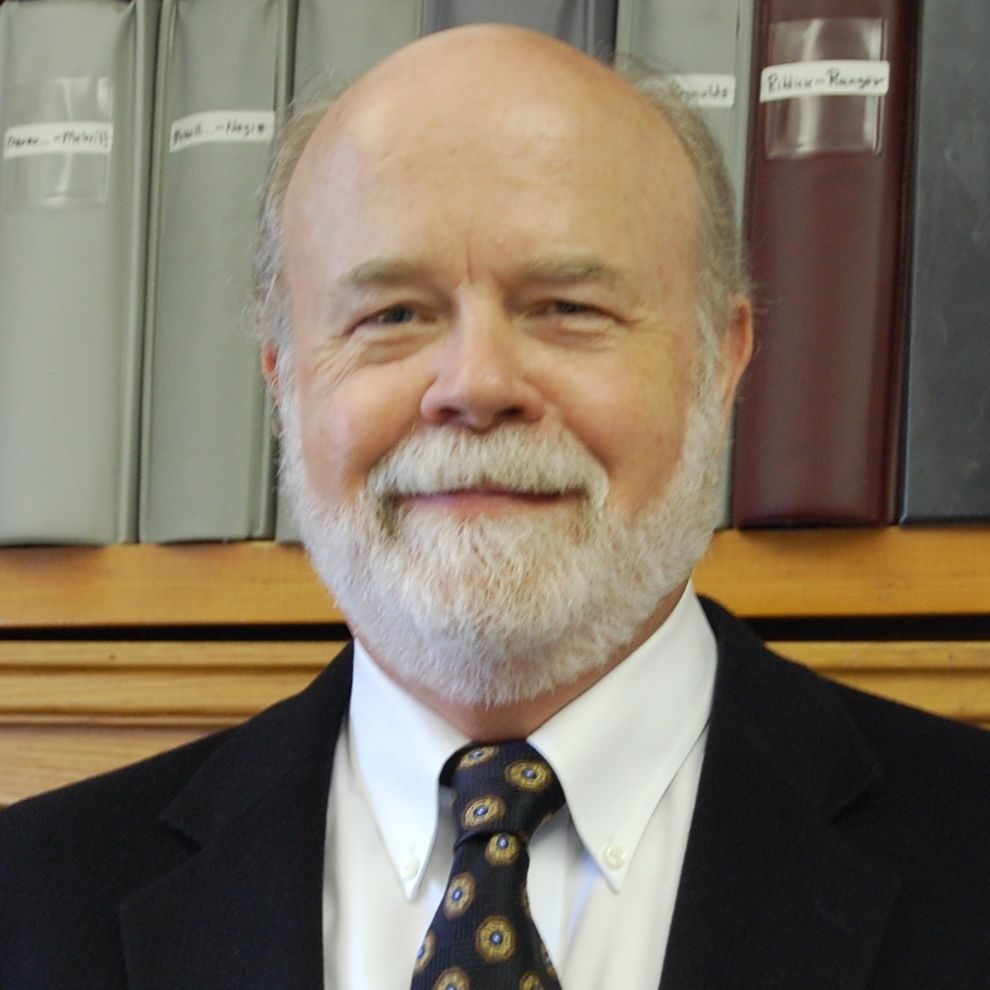
Bill Woodall
Scientific Advisor, ProcessMiner, Inc.
Bill is Professor Emeritus in the Department of Statistics at Virginia Tech. He has been active in the quality monitoring and improvement area for nearly forty years. He has published many papers on Industry 3.0 monitoring methods and some on Industry 4.0 applications. A full list of his papers can be found at www.stat.vt.edu/people/stat-faculty/woodall-bill.html. Papers are available upon request.
Email: bwoodall@vt.edu.