Over the past few decades, the manufacturing industry has gone through multiple stages of transformation aided by four industrial revolutions. Now we are on the cusp of another major revolution led by data science and artificial intelligence. Though data science is pervasive in many industries, the manufacturing industry by and large continues to make decisions based on past experiences.
However, the manufacturing industry is ripe with opportunities to utilize data science to bring operational improvement and sustainable improvement, which will reduce the consumption of raw materials, process variability, drive down costs, increase throughput and support a sustainable environment.
As an example, at ProcessMiner our research and development team has developed a data science solution that has helped an F500 and one of the largest plastic injection molding manufacturers to reduce scrap rates by more than 50 percent.
The Power of Data Science to Solve Real-World Manufacturing Problems
There is a vast amount of data available in the manufacturing industry. But many manufacturers still struggle to collect and use data due to various reasons. The major one is the presence of legacy systems.
As the first step, manufacturers must start by laying the groundwork for a safe and secure environment before they start extracting valuable data from machines as the manufacturing industry is highly prone to cyber-attacks.
Creating internal systems of data collection such as plant historians and the use of IoT to extract data from machines is perhaps the largest source of data in the manufacturing industry.
Once safe and secure systems are established to collect data, manufacturers can use data science for various applications:
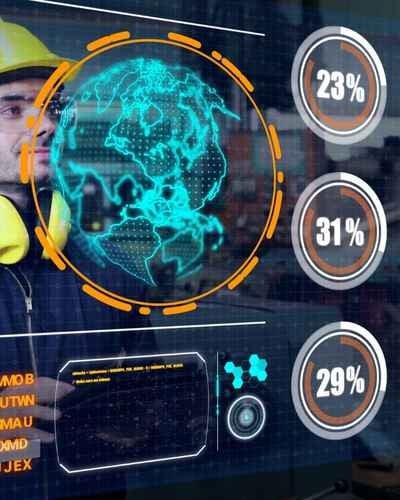
- Predictive Analytics: Predictive analytics in the manufacturing industry can be used in multiple ways – real-time monitoring of machine performance, prediction of quality of the products, and scrap reduction, to name a few.
- Preventive Maintenance: Machine breakdown and unplanned downtime are the major contributors to increasing overhead costs in manufacturing. According to some estimates, almost every factory loses at least five percent of productivity, with experiences as much as a 20% loss, due to downtime. Data science can be used to predict machine breaks in advance so that manufacturers can plan the downtime better.
- Demand Forecasting and Inventory Management: One of the basic goals of manufacturing is Just-In-Time (JIT) – Making the right products in the right quantities at the right time. Demand forecasting is an important strategy to achieve this goal. Historical data can be leveraged to forecast the demand for a product which will enable the manufacturers to manage inventory effectively.
- Supply Chain Management: Supply chain management is a complex problem to solve due to its unpredictable nature. Data science can be used to analyze vast sets of data from multiple sources such as inventory, work-in-processes, inbound shipments, present market trends, consumer sentiments, along with weather forecasts, and make optimal decisions.
The Journey Toward AI-Enabled Autonomous Optimization for Continuous Manufacturing
When ProcessMiner began the journey towards AI-enabled autonomous optimization for continuous manufacturing, we knew it was going to be a game-changer in the manufacturing industry. Fast forward to a few years later, we made a significant impact in the pulp and paper and plastics industries.
Our industry-leading autonomous control solution delivers process improvement recommendations and parameter control changes in real-time to the production line. Our platform ensures high-quality output while driving reductions in scrap, defects and waste commonly encountered in complex manufacturing processes.
Below are a couple of successful examples:
- ProcessMiner team developed a data science solution that helped an F500 plastic injection molding manufacturer to reduce scrap rates by more than 50%.
- Our artificial intelligence platform achieved unprecedented autonomous chemistry control for the tissue mill. Using a closed-loop controller in conjunction with quality parameter predictions, the mill was able to control its strength chemistry autonomously to ensure optimal chemical feed and adhere to target parameters. As a result, there was a 25% reduction in wet strength chemistry, a 33% reduction in wet tensile variation, and a 98% increase in target adherence.
There are various challenges to applying data science in manufacturing. A few of them are:
- Lack of subject matter expertise: SME knowledge is of paramount importance in business understanding, knowing the manufacturing process, and identifying the problem to be solved.
- Lack of readily usable data: The data in the manufacturing industry in most cases is not readily available to be used. It needs to be sourced from various data sources and be made available to extract insights.
- Complex nature of the manufacturing process: The data science community has achieved state-of-the-art solutions in areas such as computer vision and natural language processing. But the manufacturing process is highly complex and has temporal and spatial relationships between multiple process variables. There is a lot of scope for pushing the boundary further to achieve better results.
The manufacturing industry is undergoing a significant transformation in recent years. In this article, we have outlined how data science can potentially transform the manufacturing industry if we set up the right tools for data access and get the required subject matter expertise.
As we have demonstrated successfully, it is critical that the manufacturing industry takes full advantage of one of its most important assets, DATA. Coordinating the implementation of manufacturing process data with applied data science and domain expertise is a major lever in modernizing the production process.
Authors: Mahendra Reddy, Principal Data Scientist, and Dr. Chitta Ranjan, Director of Science, ProcessMiner
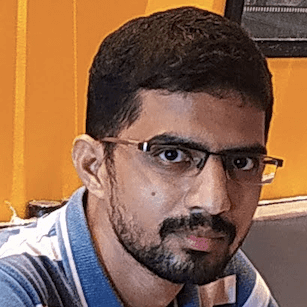
Mahendra Reddy
Principal Data Scientist, ProcessMiner
Mahendranath Reddy is the Principal Data Scientist at ProcessMiner Inc. He is an accomplished data scientist with 6+ years of experience in applied machine learning. Previously, he worked at Tiger Analytics LLP and Ericsson as a data scientist.
He graduated from the Indian Institute of Technology Madras in 2013 with a bachelor’s degree in Mechanical Engineering. While in college, he is a high achiever and he published a paper in the International Journal of Heat and Mass Transfer in March 2014 under his Professor’s guidance. While working at Ericsson, he won the ACE Award which is given to individuals for their focused and value-added contributions.
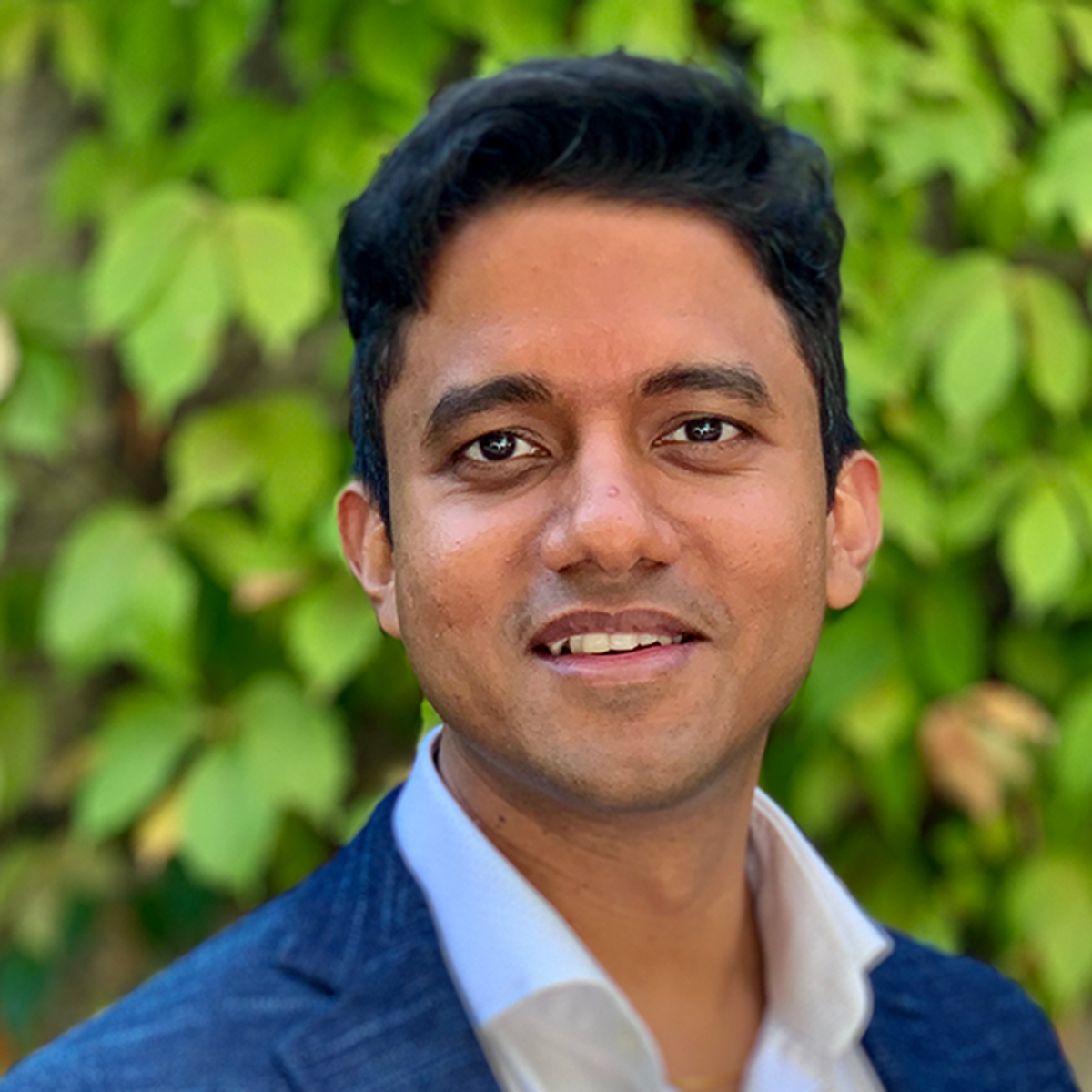
Dr. Chitta Ranjan
Director of Science, ProcessMiner
Dr. Chitta Ranjan is the Director of Science at ProcessMiner, Inc. He is author of the textbook, “Understanding Deep Learning: Application in Rare Event Prediction.” He earned his Ph.D. in Statistics from the School of Industrial and Systems Engineering at Georgia Tech. Prior to this, he completed his bachelor’s in IE from IIT Kharagpur, India.
He specializes in developing real-time AI systems. He is a recipient of best paper awards for his research. He also serves on multiple boards of directors in the analytics and engineering space. He has also worked at Pandora Media, and eBay.
Dr. Ranjan is an active contributor to the AI community and an influencer. His publicly available content on YouTube, TowardsDataScience, GitHub, Python Library (PyPi), journal publications, and other open-source contributions have over half a million readers. Globally, he has over 10k followers.